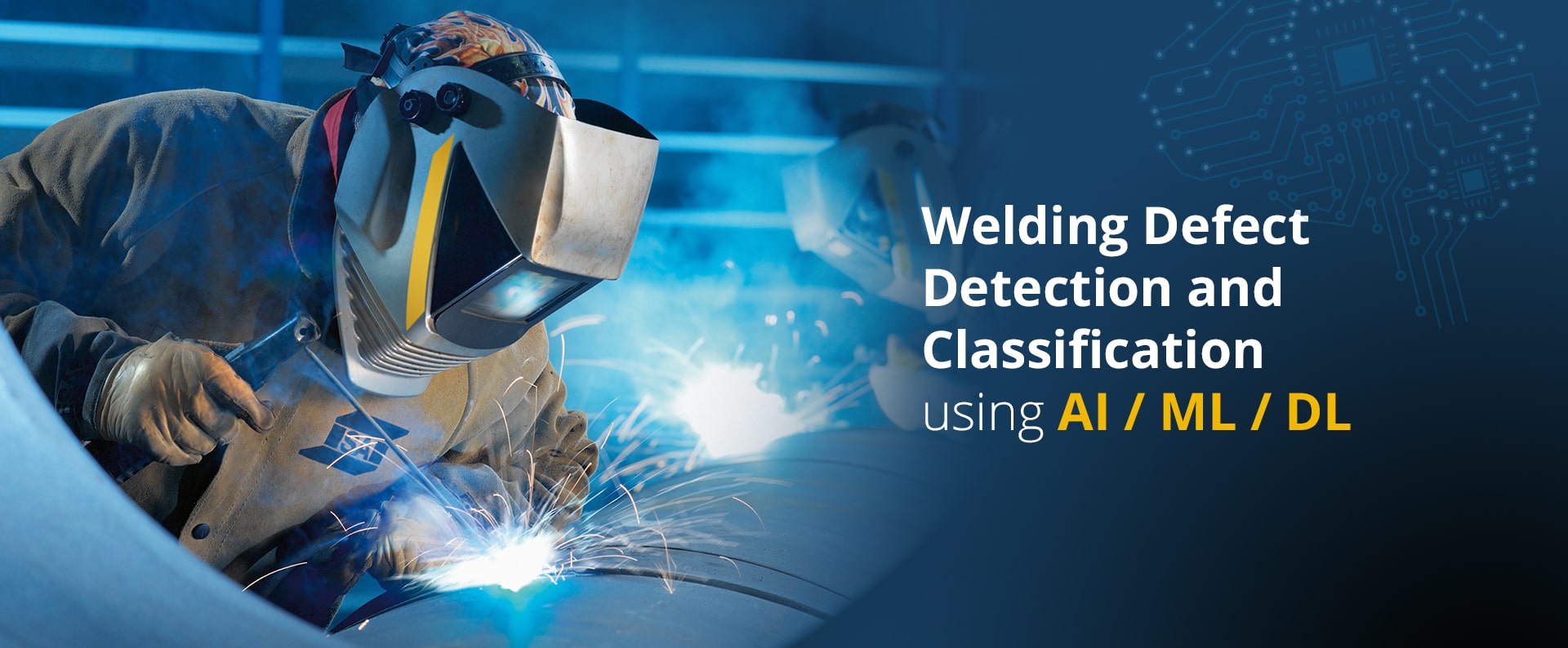
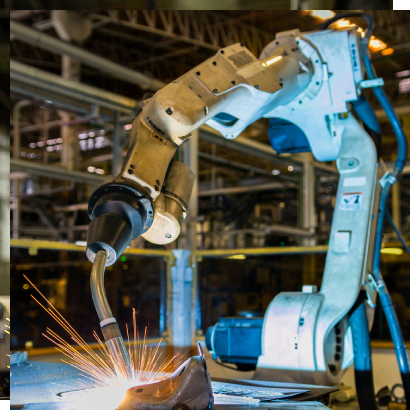
Welding Defect Analysis
Welding defects are common in the manufacturing process, and it’s impossible to form a defect-free welding joint, but the defects can be reduced to a particular extent using machine vision enabled by Artificial Intelligence and Machine Learning.
Advantages of implementing Machine Vision and Machine Learning for
defect analysis in Welding
- No more human errors
- Quality product
- Avoid damages due to defect
- Less time for quality inspection
- High accuracy in defect identification and classification
Types of Welding Defects that can be analyzed using
Machine Learning / Deep Learning
01
Porosity
in the weld zone.
02
Spatter


03
Incomplete Fusion
04
Under filling


05
Inclusion
06
Incomplete Penetration


07
Lamellar Tears
This is the main problem in low-quality steel it occurs in steel plate which has low ductility in the thickness direction which causes by non-metallic inclusions like sulphides, oxides that have been elongated during the rolling process.
08
Cracks
as longitudinal, transverse, cater, under bead and toe
cracks.


09
Slag Inclusions
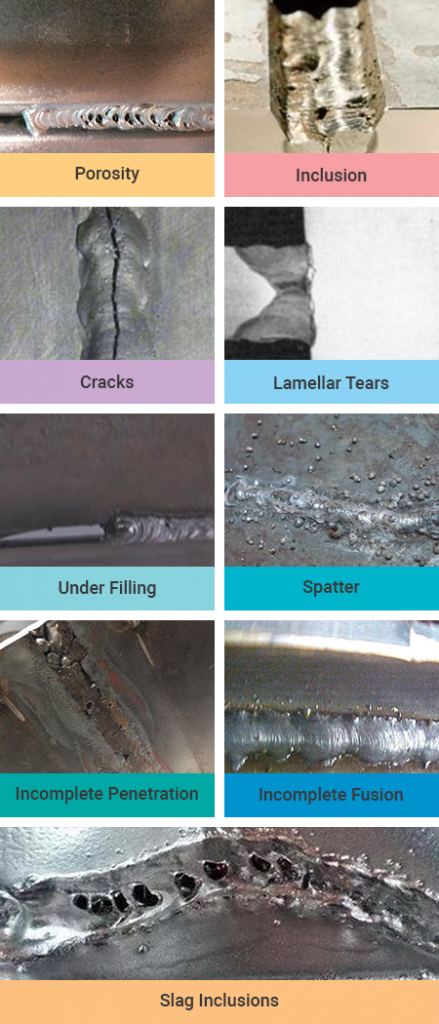
Root Causes
- Welding temperature is too high
- Welding temperature is too low
- Welding pool is too large
- Joint included angle is too low
- Electrode and torch angle is incorrect
- Welding arc is too long
- The polarity is incorrect
- Insufficient gas shielded
- Unfavourable bead position
- High electrode speed
Realtime Welding Defect
Analysis
The main defects in laser welding are lack of penetration and spatters. The root cause are Heat Input, Mismatching parts, and nozzle deviation There will be defects in automated laser welding, but checking them manually during the production in realtime is not possible. We need to have a live welding monitoring system, which will analyse the Heat temperature, Mismatching parts & Nozzle deviation in realtime to find the root cause immediately. After the production, there will be a separate visual inspection process to find the defects in the welding.
Identification and classification of welding defects using HD / X-Ray images
How we find welding defects using
machine learning?
We developed AI, Machine Learning & Deep learning Models which will use various sensor data and image data to detect anomalies in welding . To identify the root cause, specifically for the heat, we use Photo diode sensors and cameras. These data are used to train the machine learning algorithms, which will help use to find out the heat variations, nozzle deviation and the quality of the welding. Our smart cameras and image processing techniques will find out the issues real-time using metal vapor plume and splatters captured during the welding. Using these data we will calculate, the height of the plume, centroid, perimeter, welding dust and the quantity of spatters. This will help to find out the root causes immediately in the laser welding. We use state of the art AI/ ML & deep-learning technologies.
The correct and incorrect images will be collected, the visual features will be extracted using image processing from the image which will be used as input for the classifiers. We implement a deep learning system with supervised learning which will help us to achieve the best detection and high accurate defect classification.
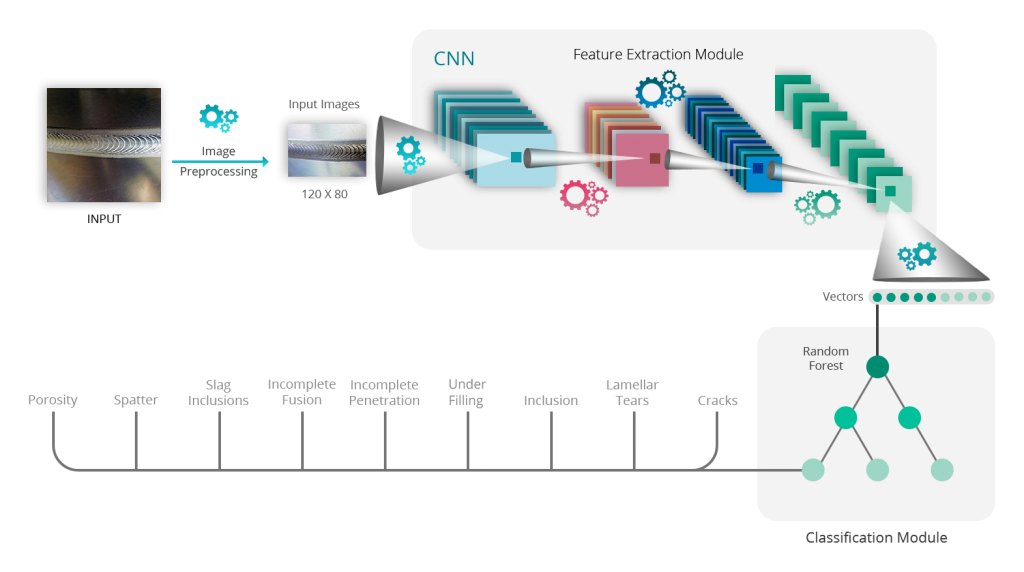
Technology Stack
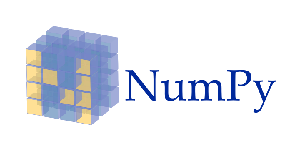
Numpy
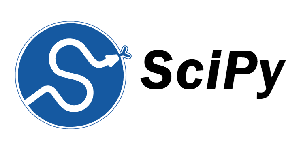
Scipy
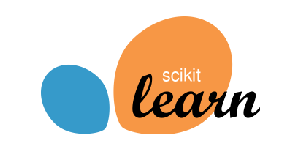
Scikit -Learn
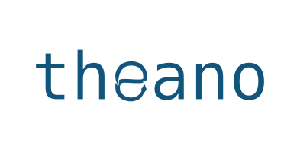
Theano
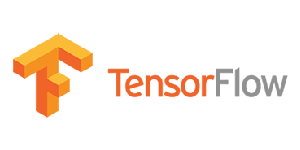
TensorFlow
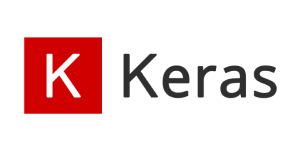
Keras
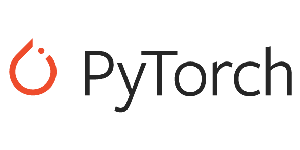
Pytorch
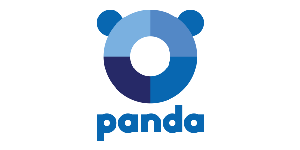
Pandas
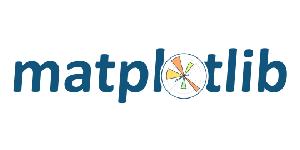
Matplotlib
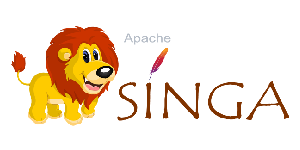